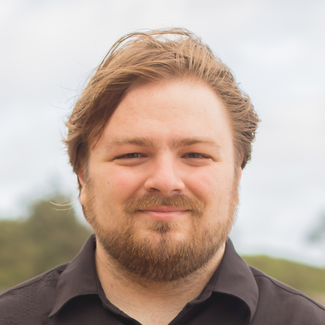
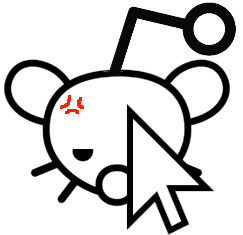
Mostly, yes. I don’t have enough other stuff to warrant storing it separately in a garage.
Also on masto: https://octodon.social/@aspensmonster
Keyoxide: https://keyoxide.org/79895B2E0F87503F1DDE80B649765D7F0DDD9BD5
Mostly, yes. I don’t have enough other stuff to warrant storing it separately in a garage.
My garage is huge compared to my house. It has 2 cars, a laundry, and all of the stuff I don’t use every day.
…
You get all the stuff into the same size house
Sounds like the problem is all the stuff.
They’re Frodo Douche Baggins. Not much point in feeding them.
The whole point of federation is that there is no “most active” one. The servers all federate with each other. I don’t have to be on lemmy.world to see its posts. The show up just fine in the All
tab on my instance.
Sincerely, leftist pacifist
Why are you a pacifist? All rights are won through violence.
As for your assertion on the origins of rights, that’s absolute bullshit. The vast majority of worker’s and other civil rights have been won via peaceful protest.
The history of society is the history of class struggle. Those rights were earned through struggle, not through asking nicely.
I want more (battery) life, fucker.
Man, there’s a lot of shit one can criticize Apple for, but planned obsolescence? I’m typing this from my 2012 MacBook Air, which has my iPhone 8 plugged into it that I use for work every day. I don’t upgrade because I don’t have a need to.
I don’t upgrade because I don’t have a need to.
You don’t upgrade because you can’t. Apple intentionally locks down its products. And that’s before getting into the numerous lawsuits over battery life and iOS slowdowns.
Apple is absolutely engaged in planned obsolescence.
Scratch a liberal and a fascist will bleed.
Scratch a liberal and a fascist will bleed.
Lemmy.world, you are positively glowing right now :3
It never ceases to amaze me how threatened liberals are by tiny groups of commies. And of course, the fact that a bunch of liberals are busy denigrating the very commies that made their migration away from capitalist Reddit possible in the first place is, unfortunately, very par for the course for liberals.
I’m excited to see federation between the two instances finally get going :D
Then there is the tankie Left, which also started with good intentions but seem to have confuse the recipe-book of slogans and the Party über alles discipline invented in the late 19th century and early 20th century by middle class intellectuals to inspired the near-illiterate masses of the time to create an utopian leftwing world (which didn’t work) with the actual thinking Principles and Intentions from which the rules were made.
The “tankies” are absolutely not utopian. There was a great big schism about this very question more than a century ago, with Marxists roundly rejecting the utopianism of the libertarian socialists (Anarchists). Socialism: Utopian and Scientific by Engels is a good starting point.
but I hate it when someone behind the scenes decides whether or not I should be able to debate/talk to my political opposites.
Then you’re not gonna be a fan of federated alternatives like Lemmy. At least unless you run your own instance. Because instance admins can defederate from other instances, preventing you from reaching those defederated instances. In my mind, this is a Good Thing. I don’t want to federate with nazis and fascists myself, and I want to minimize my association with anyone who does. If “having a dialogue” with people that want us dead is super important, then they can pick a different instance to call home.
I wish we had a Parenti bot. Nonfalsifiable Orthodoxy etc etc.
During the cold war, the anticommunist ideological framework could transform any data about existing communist societies into hostile evidence. If the Soviets refused to negotiate a point, they were intransigent and belligerent; if they appeared willing to make concessions, this was but a skillful ploy to put us off our guard. By opposing arms limitations, they would have demonstrated their aggressive intent; but when in fact they supported most armament treaties, it was because they were mendacious and manipulative. If the churches in the USSR were empty, this demonstrated that religion was suppressed; but if the churches were full, this meant the people were rejecting the regime’s atheistic ideology. If the workers went on strike (as happened on infrequent occasions), this was evidence of their alienation from the collectivist system; if they didn’t go on strike, this was because they were intimidated and lacked freedom. A scarcity of consumer goods demonstrated the failure of the economic system; an improvement in consumer supplies meant only that the leaders were attempting to placate a restive population and so maintain a firmer hold over them.
If communists in the United States played an important role struggling for the rights of workers, the poor, African-Americans, women, and others, this was only their guileful way of gathering support among disfranchised groups and gaining power for themselves. How one gained power by fighting for the rights of powerless groups was never explained. What we are dealing with is a nonfalsifiable orthodoxy, so assiduously marketed by the ruling interests that it affected people across the entire political spectrum.
― Michael Parenti, Blackshirts and Reds: Rational Fascism and the Overthrow of Communism
The United States is a deeply unserious country.
Fortunately, I’m not a liberal, so I’ve got no problem readin’ 'em.
If “lemmy is developed by socialists/communists/leftists” is enough for folks to steer clear of it (or move over to kbin, as I’ve seen at least one radlib insist that folks do rather than lemmy), then… “task failed successfully” in my eyes. No real loss.
The associated paper and its abstract:
Recent work claims that large language models display emergent abilities, abilities not present in smaller-scale models that are present in larger-scale models. What makes emergent abilities intriguing is two-fold: their sharpness, transitioning seemingly instantaneously from not present to present, and their unpredictability, appearing at seemingly unforeseeable model scales. Here, we present an alternative explanation for emergent abilities: that for a particular task and model family, when analyzing fixed model outputs, one can choose a metric which leads to the inference of an emergent ability or another metric which does not. Thus, our alternative suggests that existing claims of emergent abilities are creations of the researcher’s analyses, not fundamental changes in model behavior on specific tasks with scale. We present our explanation in a simple mathematical model, then test it in three complementary ways: we (1) make, test and confirm three predictions on the effect of metric choice using the InstructGPT/GPT-3 family on tasks with claimed emergent abilities, (2) make, test and confirm two predictions about metric choices in a meta-analysis of emergent abilities on BIG-Bench; and (3) show how similar metric decisions suggest apparent emergent abilities on vision tasks in diverse deep network architectures (convolutional, autoencoder, transformers). In all three analyses, we find strong supporting evidence that emergent abilities may not be a fundamental property of scaling AI models.
Page two of the paper states their thesis pretty succinctly:
In this paper, we call into question the claim that LLMs possess emergent abilities, by which we specifically mean sharp and unpredictable changes in model outputs as a function of model scale on specific tasks. Our doubt is based on the observation that emergent abilities seem to appear only under metrics that nonlinearly or discontinuously scale any model’s per-token error rate.
And figure two walks through the argument for it concisely as well:
(OCR for image: Figure 2: Emergent abilities of large language models are creations of the researcher’s analyses, not fundamental changes in model outputs with scale. (A) Suppose the per-token cross-entropy loss decreases monotonically with model scale, e.g., LCE scales as a power law. (B) The per-token probability of selecting the correct token asymptotes towards 1 with increasing model scale. © If the researcher scores models’ outputs using a nonlinear metric such as Accuracy (which requires a sequence of tokens to all be correct), the researcher’s measurement choice nonlinearly scales performance, causing performance to change sharply and unpredictably in a manner that qualitatively matches published emergent abilities (inset). (D) If the researcher instead scores models’ outputs using a discontinuous metric such as (Multiple Choice Grade, which is similar to a step function), the researcher’s measurement choice discontinuously scales performance, causing performance to change sharply and unpredictably in a manner that qualitatively matches published emergent abilities (inset). (E) Changing from a nonlinear metric to a linear metric (such as Token Edit Distance), model shows smooth, continuous and predictable improvements, ablating the emergent ability. (F) Changing from a discontinuous metric to a continuous metric (e.g. Brier Score) again reveals smooth, continuous and predictable improvements in task performance, ablating the emergent ability. Consequently, emergent abilities may be creations of the researcher’s analyses, not fundamental changes in model family behavior on specific tasks.)
But alas, AI/ML isn’t my wheelhouse, so the paper quickly starts to go over my head.
Looks to me like
lemmy.world
still haslemmy.ml
andlemmygrad.ml
linked. I can see this post onAll
at least.